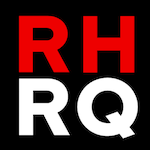
Red Hat Research Quarterly
Power surge: the push for sustainability in high-performance computing and AI workloads
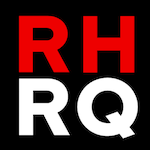
Red Hat Research Quarterly
Power surge: the push for sustainability in high-performance computing and AI workloads
John Goodhue has perspective. He was there at the birth of the internet and the development of the BBN Butterfly supercomputer, and now he’s a leader in one of the toughest challenges of the current age of technology—sustainable computing. Comparisons abound: one report says carbon emissions from cloud computing equal or exceed emissions from all […]
Article featured in
John Goodhue has perspective. He was there at the birth of the internet and the development of the BBN Butterfly supercomputer, and now he’s a leader in one of the toughest challenges of the current age of technology—sustainable computing. Comparisons abound: one report says carbon emissions from cloud computing equal or exceed emissions from all commercial flights combined. Another suggested that by 2027 AI workloads could be using as much energy as a country the size of the Netherlands. That leaves computing and research communities with a conundrum: how do we solve real-world global challenges without worsening climate change or devastating power grids?
RHRQ asked Han Dong and Parul Singh, engineers working on open source projects related to energy efficiency, to talk with John about his work as director of the Massachusetts Green High-Performance Computing Center (MGHPCC), a joint venture of Boston University, Harvard, MIT, Northeastern, and the University of Massachusetts system. The MGHPCC provides computing and storage resources for over 20,000 faculty and student researchers and educators, including the MOC Alliance-supported New England Research Cloud (NERC) and the Red Hat Collaboratory at Boston University, and works to maximize energy efficiency while minimizing environmental impacts. They offer us a deep dive into the factors that bear on sustainability, from renewable energy sources and hardware choices to scheduling and tuning policies. The latter two, by the way, are the subject of Han and Parul’s article on the PEAKS project, also in this issue.
—Shaun Strohmer, Ed.
Parul Singh: Tell us about your background in hardware. How did that lead you to eventually running the MGHPCC?
John Goodhue: Coming out of school, I ended up spending equal time on networks and computing. It was an exciting time for networking technology, with the technologies that became the foundations for the Internet just coming into play. At the same time, the company I was working at (BBN) was working on tiny networks that fit in a cabinet, interconnecting a large number of microprocessors to form a single computer system. That work led to the BBN butterfly, the second or third generation of the thing that we now call a cluster.
In 2010, the world was just beginning to realize that energy efficiency in datacenters might matter
I ended up at the MGHPCC after leaving a startup in the high-performance computing business. It’s obviously a very different thing: you can think of it as level minus one in the OSI (Open Systems Interconnection) stack. At the time we started to build it in 2010, the world was just beginning to realize that energy efficiency in datacenters might matter. Before then, even the idea of turning your air conditioners off in the winter was kind of a “why bother.” That’s how far the dark ages people were. We drove pretty hard on energy efficiency, and our timing was kind of lucky that way.
Parul Singh: From your perspective, what got people to start thinking about sustainability in computing?
Developers of datacenters are under great pressure to get the thing built and into operation so they can start to get return on investment. Being thoughtful about energy efficiency and environmental footprint often takes a back seat as a result.
John Goodhue: There was a turning point in the thinking in the industry around 2005, exacerbated by the recession and also influenced by growing awareness of the need to pay attention to climate change. An early challenge was finding ways to work with our architects and engineers to take steps that seemed radical at the time but are fairly commonplace. Though even today, developers of datacenters are under great pressure to get the thing built and into operation so they can start to get return on investment. Being thoughtful about energy efficiency and environmental footprint often takes a back seat as a result.
Going forward, we are beginning to see people think about what’s going to happen when three things converge. First, you’re seeing a dramatic increase in the amount of power consumed by datacenters, which is estimated to be as much as 3% of total worldwide energy demand, and may double in the coming years. Second, you’re seeing large segments of the rest of the economy pivoting to electricity. The transportation sector and electric cars are a good example, but it’s happening everywhere. Third, there’s the introduction of renewables, which will make supply more intermittent.
The MGHPCC is lucky—maybe there’s a combination of skill and luck—that we settled ourselves in a city (Holyoke, MA) whose municipal electric company delivers 100% green energy to us through hydroelectric power. But they’re also forward-looking and interested in creative ways to make sure that the energy supply we receive has as light a footprint on the grid as possible. If you look out maybe 10 years, you may see, for example, battery storage as a factor. Our load is steady 24/7, but maybe we can lighten that load during peak hours and then make it heavy during non-peak hours in a managed way that potentially both saves money and offsets the impact of renewables. Tracking those types of change is a challenge that all datacenter operators face.

Han Dong: One thing we don’t really know in the systems field is how power purchase agreements work with datacenters. Can you talk about that?
John Goodhue: The energy markets are a fascinating thing. They were developed when deregulation happened and transmission, generation, and delivery were split into three different things. The MGHPCC is dealing with the municipal electric company, which was grandfathered into being able to do all three. You don’t get a discount for consuming more—or less, for that matter. They currently encourage us to have a load that’s steady with the ability to drop or decrease if there’s advantage to doing so.
If you look at the transmission rate, it’s based on monthly peak consumption. There’s an advantage to shaving peaks that accrues to our supplier. We need to have a piece of the agreement that incentivizes us to do that. If you look at something called the forward capacity charge, that’s based on your annual peak usage. That leads to a double incentive during one month of the year, but nobody knows what month that is until the end of the year. If you go to the ISO New England website, there is endless material on how the markets work. It’s remarkable that the grid and energy markets work as well as they do given the complexity of the market and the engineering that supports them.
Han Dong: You mentioned the MGHPCC is 100% renewable. What does that mean?
John Goodhue: I like to say there are three ways of viewing it. First, where do the electrons come from? A quantum physicist would say who knows—everywhere. The more practical view is the electrical engineering view. There is a dam on the Connecticut River that generates way more energy than what we consume. It is connected to the same power substation that our primary power feed is connected to. From an electrical engineering point of view, you can’t get any more tightly coupled than that to a renewable source. And there’s the added benefit that we’re not relying on transmission lines that have other environmental impacts. It’s all right there within a half mile. The third view is the market view, where renewable energy like hydro or solar energy carries a premium. So we also pay the price premium for renewable energy. At both an electrical engineering level as well as the market level, we are 100% carbon free.
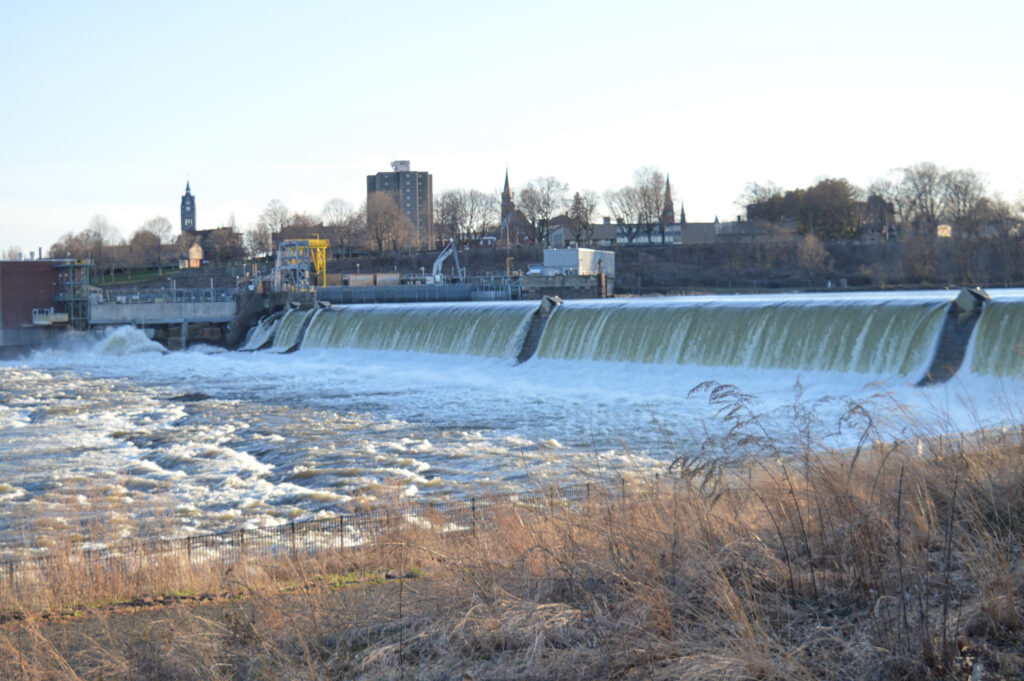
Han Dong: Is it reasonable to consider renewables free energy?
John Goodhue: It is absolutely not free. There are capital costs and maintenance costs. The only difference is that you’re not burning fuel from Texas or Pennsylvania or the Middle East. The upfront costs can be higher. The good news is that solar is getting to a point where the lifetime cost of delivering a kilowatt hour of renewable energy continues to decline, but “free” is not a description you would attach to any energy source.
Han Dong: When you say upfront costs, are you talking about the embodied carbon that’s attached to some of these sources that we don’t really think about?
John Goodhue: There are things that emit carbon and there’s things that don’t, and the money the MGHPCC pays for electricity generation goes to places that don’t emit carbon.
Han Dong: At an NSF Workshop last month, one of the issues discussed was embodied carbon in terms of datacenter servers. The standard longevity, at least for hyperscalers, is that these servers last at most about six years and then they replace them. There’s a bigger push towards prolonging the life of some of this older hardware. How do we do that?
John Goodhue: That happens at a few different levels. First, lifetimes started to increase around 2005. Before then, CPU clock rates were steadily increasing from about the late 1980s through the early 2000s, and the performance improvements in every three-year cycle were significant enough to justify replacing the server.
That equation changed as clock rates hit the wall at about three megahertz. As a result, lifetimes are now closer to five and six years—at least that’s what we’ve been seeing. GPUs are an exception, as architectural changes introduce compelling improvements every two years or so. But even there you can keep the older ones around, to support less performance-sensitive workloads for them, and the motherboard, at least, has to change.
Han Dong: What are some examples of the architectural changes that are driving GPU performance?
John Goodhue: Many of them have to do with AI workloads, which can use fixed-point arithmetic, and algorithm improvements have made it possible to use much lower predictions. It’s a complete U-turn compared to the drive for better floating-point performance needed for the scientific simulation applications.
Parul Singh: When I was working at Boston University, I learned that the MGHPCC datacenter is cooled naturally. Could you say more about how that’s done, using water and the cold temperatures in the New England area?
John Goodhue: I’ll unpack that a little bit. The cooling system for the datacenter really operates in three stages. We circulate cold water—not extremely cold, about 65 degrees Fahrenheit— into the computer room and run it through heat exchangers that either remove heat from the air that’s ejected by the servers or remove heat through water that circulates right next to the chips. The second stage transfers heat to a different water loop that circulates through a set of cooling towers. The cooling tower uses two kinds of processes to remove heat from the water that circulates through them. One is evaporation—evaporation is a cooling process, as we learned in elementary school—and the other is, say in January, it’s just cold outside so the water cools down.
The chillers are the biggest energy hogs in the building, so we operate to minimize the use of that resource.
There are two ways of moving the heat from the computer center loop to the cooling tower loop. Most of the year, we just use a heat exchanger because the water from the cooling tower loop is cold enough. There are times during the year in Massachusetts when it’s too hot outside to allow us to cool the water enough using just heat exchangers. In that case, we use chillers, which are just refrigeration units that move heat from one place to another. The chillers are the biggest energy hogs in the building, so we operate to minimize the use of that resource. As I mentioned earlier, most datacenters didn’t bother to minimize the use of chillers until the early 2000s, when datacenters started to get more energy conscious.
Han Dong: None of this information you’re talking about is exposed to a user. Is there any value in getting this information to an end user? If there’s a way to let someone know before they deploy their job that the cooling costs are likely to be high at a given time, is it worth incentivizing them to think, “Maybe I shouldn’t run my heavy workload today because of the potential cooling costs?”
John Goodhue: I’ll start with an analogy. I’ve spent a lot of time building networks, and one of the key reasons the internet works at all is layering, right? When I send a packet with a source and a destination address in a checksum using IPV4, I don’t need to know anything about what happened next except whether the packet was dropped. Even that layer doesn’t tell me how to figure it out. There’s the layer above that, which works on end-to-end reliability when it’s needed, or maybe it’s tolerant of dropping packets on the way up the stack in the session that we’re running. If every layer had to know what was happening in every other layer, you could never change it and it would frequently break.
The analogy isn’t perfect but it holds. There are signals the grid can give our energy supplier that our energy supplier can pass through to us about peak demand, for example, and backing off when a multi-peak happens. It’s feasible to pass those signals through, but you would need to react quickly. There is some promising research at BU and other universities looking at how to do that while minimizing impact on performance.
It is also possible to react to a signal at a lower level in the stack, by lowering the CPU clock rate. This has the advantage of being invisible to the application software, other than a decrease in performance.
Han Dong: The operating system has mechanisms to do exactly what you’re talking about. It just doesn’t have the policies there right now.
Let’s address the question everyone’s concerned about. From your perspective as director of a high-performance computing facility, what are the biggest challenges presented by generative AI and LLMs? For example, in terms of workload, how much of the work in MGHPCC consists of things like generative AI and large language models? I’m assuming it’s increasing, right?
John Goodhue: It is increasing. If I use GPU installations as a proxy, it’s growing substantially. The reason I can’t say exactly is there’s been a wave of installations with people who put in orders last year but supply chain problems kept them from actually getting their stuff until the last three months or so, so the systems are just beginning to come up.
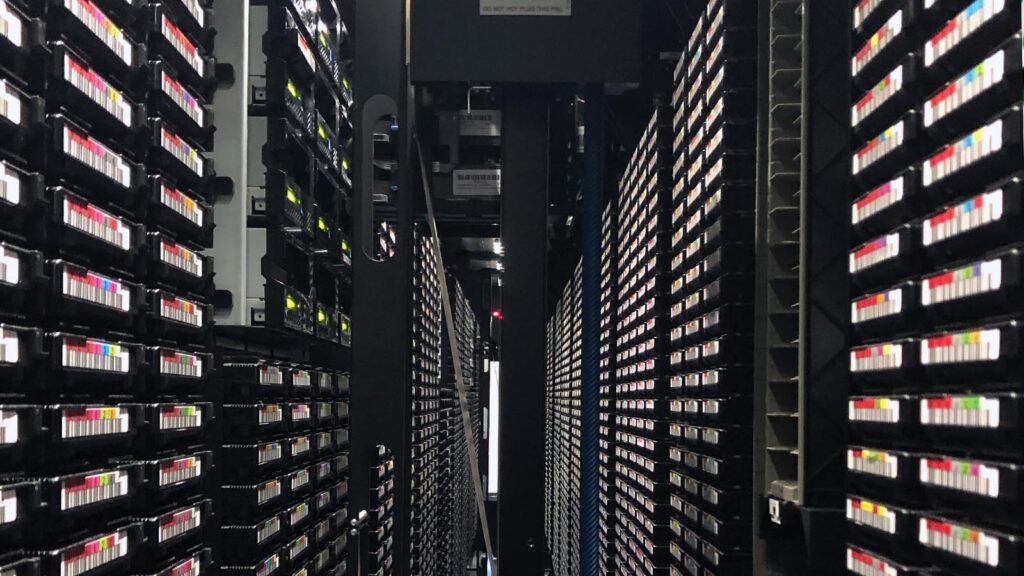
Han Dong: Are a lot of them doing training jobs right now or are they also setting up inferencing servers on the MGHPCC?
John Goodhue: That’s a good question. I suspect that there tends to be more training, compared to industrial workflows, but I don’t know what the mix is.
Han Dong: Are you thinking about how the MGHPCC might adapt? Do you have to change how you think about the cooling and layout of the datacenter?
John Goodhue: The MGHPCC has been able to take this change in stride, in part due to early design decisions, and in part because we started supporting systems with high power density in 2016, well before the AI wave appeared.
Not much has changed with the AI boom, with one exception, which is the amount of power that gets consumed per square foot. Ten years ago, high-end enterprise workloads were six kilowatts per rack (KPR), and research computing workloads were probably more like 12 KPR. GPU-heavy research computing workloads can now be as much as 60 or 70 KPR, going up to 100 KPR. So we are seeing the amount of computing resources per square foot increase by factors of two and three. How we distribute power hasn’t changed our cooling much at all.
The technology is evolving rapidly, and it’s essential that research computing and datacenters do not fall behind their for-profit counterparts.
More broadly, the Coalition for Academic Scientific Computation (CASC) has organized several working groups around the topics of AI, energy efficiency, and building and maintaining datacenters like the MGHPCC. The technology is evolving rapidly, and it’s essential that research computing and datacenters do not fall behind their for-profit counterparts.
Parul Singh: Thanks for your time, John. This has been quite interesting and very helpful.
SHARE THIS ARTICLE
More like this
Václav Matyáš, Professor with the Centre for Research on Cryptography and Security at the Faculty of Informatics at Masaryk University.
It is by now well understood that we humans are capable of creating systems that are more complex than we can understand.
Since the inception of artificial intelligence research, computer scientists have aimed to devise machines that think and learn like human beings. What else could AI do?
Thoughts on open source and open collaboration from the Greater Boston Research Interest Group (RIG).
Research at Devconf.us: Optimizing and automating the foundations of computing.
Parallelism promises to make programs faster, yet it also opens many new pitfalls and makes testing programs much harder.
To design effectively for our users, we need to learn more about them. If we don’t, we may make a product that our users can’t be efficient in, or worse, a product that our users have no need for in the first place.
The recent advances in AI and telecommunications are enabling a new set of complex cyber-physical systems, including those for safety-critical applications.
I have been spending a lot of time lately thinking about all the hard problems involved in managing large-scale systems. Why? Well, it turns out to be a really important topic for Red Hat Research and for the Red Hat engineering community that we hope to serve.